Understanding how to innovate in clinical trial design
WASHINGTON, D.C.—Randomized clinical trials (RCTs) are the gold standard for testing medical interventions. However, researchers are using multiple new and creative ways to design these trials to account for real-world scenarios and produce information relevant to practicing clinicians. At ACR Convergence 2024, the session Innovative Clinical Trials: Precision, Design and Optimization provided tremendous insights into these important topics.
Heterogeneity of Treatment Effects
The first speaker, Kelli D. Allen, PhD, professor of medicine, research health scientist, Durham VA Medical Center, University of North Carolina at Chapel Hill, spoke about intervention optimization and heterogeneity of treatment effects (HTE). HTE refers to when a treatment has different effects on different individuals or subgroups within a study population. Quantitative subgroup interactions refers to a large improvement for some patients and little to no improvement for others. Qualitative subgroup interactions describes when one treatment may work better for one subgroup and the other treatment may be better for another subgroup.
Dr. Allen noted that such heterogeneity is common in clinical trials, and, knowing that often groups of patients do not respond to a specific intervention or treatment, researchers ought to plan for what they should do for these patients. For this, researchers can use adaptive interventions. An example of this approach is from a study conducted by Dr. Allen et al. on a stepped exercise program for patients with knee osteoarthritis (OA). The initial intervention was an internet-based exercise program, and participants were assessed for clinically relevant improvement in pain and function after three months. For those patients who did not benefit, telephone-based physical activity coaching was added to the exercise program. If still no benefit was seen with this additional intervention after three months, then physical therapy visits were implemented.1
Dr. Allen explained that HTE can affect analysis of results, and this issue can be addressed through different strategies meant to provide individual predictions of treatment effects that take into account multiple relevant characteristics simultaneously. Risk modeling involves developing a multivariable model that predicts risk for an outcome and applies that model to stratify patients within a trial to examine risk-based variation in treatment effects. Effect modeling uses a regression model developed from trial data to understand how much of the average effect is modified by baseline predictors.
Data-driven approaches are also used, and these employ technologies, such as machine learning, that take advantage of all the rich data collected in a trial. Dr. Allen referenced the qualitative interaction tree (QUINT) method that can identify subgroups of study patients for whom treatment effect differs significantly based on the characteristics of those patients. Using QUINTS may both help identify which patients are most likely to benefit from a specific intervention based on characteristics unique to them, such as body mass index in patients with knee OA, and may help with the design of future clinical trials, where patients may be randomized based on specific characteristics.
The main thrust of Dr. Allen’s talk was that HTE is real and widely prevalent. Thus, researchers must be creative in accounting for this heterogeneity.
Multiphase Optimization Strategy
The second speaker was Sandra Soto, PhD, MPH, BSN, assistant professor in the School of Nursing and Thurston Arthritis Research Center at the University of North Carolina at Chapel Hill. Dr. Soto began by describing the classical approach for developing interventions, in which randomized clinical trials test multiple intervention components simultaneously in one package and investigators piece together their best intervention based on evidence from existing literature, theory and experimentation.
Dr. Soto provided the example of modifying an existing program meant to enhance physical activity among patients with arthritis. Using the classical approach, researchers may choose to add multiple interventions, such as use of pedometers, encouragement through social networking, phone calls from community health workers and creation of an educational video series. Ultimately, these interventions may be found to be effective, but how can a researcher know which components were most or least helpful? For this, the Multiphase Optimization Strategy (MOST) comes in handy.
The first step in MOST is preparation, which refers to laying the groundwork for optimization by deriving a conceptual model, selecting candidate intervention components, conducting pilot work and identifying optimization objectives. The next step is optimization, during which such approaches as factorial design can allow for individual intervention components to be turned on or off, such as only giving pedometers or using calls from community health workers for some, but not all, participants, and comparing the main effects of each component, as well as understanding interactions between components. The third step is to ask: Is the optimized intervention expected to be sufficiently effective? This can be evaluated by looking at P values, effect size, cost, time or other measures of relevance.
The final phase is evaluation, in which researchers can confirm the effectiveness of the optimized intervention. MOST is meant to allow researchers to be methodical in how they assess and optimize interventions, thereby being able to separate the wheat from the chaff.
More & more, clinicians have a growing number of possible treatments available to choose from, but lack evidence to guide those decisions.
Sequential Steps
The session’s final speaker was Laura Schanberg, MD, MACR, professor of pediatrics, Division of Pediatric Rheumatology, Duke University School of Medicine, Durham, N.C. Dr. Schanberg described how clinicians routinely make a series of treatment decisions with patients over the course of their disease with the goal of achieving the most beneficial expected outcome. Along the way are key decision points, milestones in the disease process, events that prompt the need for specific decisions and several feasible treatment options at each decision point.
More and more, clinicians have a growing number of possible treatments available to choose from, but lack evidence to guide those decisions. This situation prompted the development of the Sequential, Multiple Assignment, Randomized Trial (SMART), in which sequential steps are adapted to a patient’s individual response, subjects are randomized to feasible options at key decision points in which equipoise is achieved, and evolving characteristics—beyond just baseline characteristics—are collected between and at decision points.
To illustrate this model, Dr. Schanberg discussed a trial that would seek to treat patients with juvenile idiopathic arthritis who have had inadequate response to a tumor necrosis factor (TNF) alpha inhibitor. At decision point #1 (i.e., at initial TNF-alpha failure), the participant can either be treated with a different TNF-alpha inhibitor or receive medication with a different mechanism of action. At decision point #2 (i.e., after 3–6 months of the initial treatment), responders could be continued on treatment and non-responders could be switched to a new non-TNF-alpha biologic.
The SMART design for a clinical trial is meant to mimic what which would occur in real-life and create a plan for how patients within a trial will be treated depending on their course and response—or lack of response—to a treatment within a trial.
Dr. Schanberg noted several potential benefits to SMART design of clinical trials, including the ability to target a range of scientific questions, the ability to use smaller sample sizes of participants and still yield important outcomes data, and yield scientific insights that are highly relevant to clinical practice.
In Sum
The session was full of wisdom for those willing to modify classic models of RCT design. With more talks like these, the RCT space is likely to continue to evolve—and improve—in the coming years.
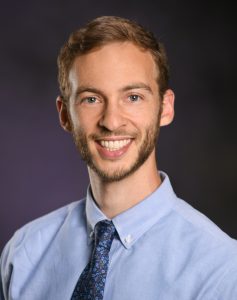
Dr. Jason Liebowitz
Jason Liebowitz, MD, is an assistant professor of medicine in the Division of Rheumatology at Columbia University Vagelos College of Physicians and Surgeons, New York.
Reference
- Allen KD, Woolson S, Hoenig HM, et al. Stepped exercise program for patients with knee osteoarthritis: A randomized controlled trial. Ann Intern Med. 2021 Mar;174(3):298–307.