She shared the example of a study of heart failure patients at Duke, in which researchers assumed that most patients received care at that institution. Researchers identified 20 distinct payers and noted that 80% of patients were covered by nine payers. “This gives you a sense of the linkage that may be required,” she said.
Linkage using claims data also ignores uninsured patients, who may have complex health issues.
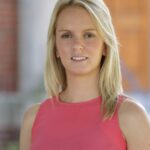
Dr. Bradley
Choosing fit-for-purpose data sources, managing treatment changes over time and considering relevant risk windows are also important considerations to optimize post-marketing safety assessments, said Marie Bradley, PhD, MPharm, MScPH, senior epidemiologist with the Division of Epidemiology, Office of Surveillance and Epidemiology, Center for Drug Evaluation and Research with the FDA.
Like Dr. Curtis, Dr. Bradley shared some of the benefits and risks associated with various data sources, such as EHRs, insurance claims and registries. For instance, registries may be rich in clinical data but often have small, highly selected patient groups and a lack of comprehensive data on drug use.
EHRs may contain more accurate information on diagnoses than other data sources and often contain data on smoking status or body mass index. Body mass index may be an important confounder in, for example, studies examining certain cancers or diabetes as outcomes.
Connecting Outcomes to Treatments
Deciding how to handle patients who switch treatments after initiation is another concern, Dr. Bradley said. This often arises for rheumatology treatments, and there are a few possible ways to manage this in a study. Using an as-treated approach, researchers can censor or suppress data from patients who switch treatments to ensure that only outcomes occurring while the patient is on the study treatment are attributed to the drug. This avoids questions about whether a new outcome is associated with the initial treatment or as a result of a newer treatment. One challenge to keep in mind is that a large degree of censoring can lead to selection bias, sample size concerns and a study population restricted to those likely to do well on the treatment (i.e., those likely to have a good response and no adverse events).
Another approach is intention to treat, which does not raise selection bias issues but could lead to increasing misclassification of exposure over time. In a prospective randomized study, this approach includes all participants in the statistical analysis; they are analyzed according to the group they were originally assigned, regardless of what treatment (if any) they received.