2022 ACR-FDA SUMMIT—A better understanding of premarketing safety of treatments through dose exploration and controlled studies, as well as long-term safety in post-marketing studies, could assist rheumatoid arthritis (RA), psoriatic arthritis and other rheumatology programs in the future, according to Nadia Habal, MD, Division of Rheumatology and Transplant Medicine, Office of New Drugs, U.S. Food & Drug Administration (FDA).
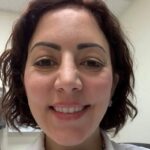
Dr. Habal
Dr. Habal and other experts addressed regulatory considerations for long-term safety assessment in clinical studies during the 2022 ACR-FDA Summit held virtually in May.
The availability of multiple, highly effective disease-modifying anti-rheumatic drugs that can help patients achieve remission or lower disease activity raises questions about the utility of long-term, placebo-controlled trials. Having patients receive suboptimal treatment for long time periods in studies is no longer appropriate, Dr. Habal said.
Traditional Study Design
Historically, treatments have been evaluated with short proof-of-concept and dose-ranging studies, followed by phase 3, confirmatory, controlled studies with 6- or even 12-month placebo-controlled periods. Studies have evolved to incorporate provisions for rescue when patients’ conditions devolve; they are transferred to alternative therapies or the active drug instead of placebo. These studies may be followed by long-term extension studies, which are often open-label and uncontrolled.
This development paradigm presents challenges for the accurate assessment of long-term safety and efficacy of the investigational treatment, Dr. Habal explained.
“While randomized comparisons are generally reliable for assessment of short-term safety, a study design with rescue and crossover between treatment arms, and uncontrolled extension studies, complicates the longer term safety assessment,” she said.
Evaluating patients at later time points is important in these studies given the unique and latent toxicities of chronic immunosuppressives, including serious and opportunistic infections, tuberculosis, malignancy, cardiovascular morbidity and mortality.
The typical RA study program has included 3,000 to 4,000 patients. However, most of the treatment exposure in these programs is during the uncontrolled portion of the study, making it difficult to evaluate whether adverse events are attributable to study drugs or other factors.
Clinical programs in RA can be optimized to help address these challenges without increasing the size of the study population or the length of exposure, Dr. Habal said. For example, robust dose exploration can better inform the risk–benefit profile of a product and the doses to be studied in the larger phase 3 program. In addition, study design should address the importance of a controlled long-term assessment, such as using an active comparator that allows for meaningful interpretation of the long-term safety and efficacy of the investigational product.
Researchers should also make sure to include a systematic plan to assess safety within a trial, just as they do for efficacy.
“A safety database with controlled data on 1,000 to 1,500 patients exposed to the proposed market dose of a new molecular entity for at least one year would be informative and feasible without the need for more patients,” Dr. Habal said. Use of pre-specified analyses for safety, particularly for adverse events of special interest, may also help address some of the uncertainties with data interpretation.
Data Sources to Assess Safety
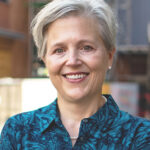
Dr. Curtis
When choosing the right data sources to assess safety, researchers may consider electronic health records (EHRs) or claims data, among other options. Each option comes with its own benefits and challenges, said Lesley Curtis, PhD, chair of the Department of Population Health Sciences, Duke University, Durham, N.C. The decision requires thinking about the purpose for each data source, how rich the data may be for a study’s purpose and the challenge of data standardization.
EHRs from a single health system may appear useful on the surface but can be limited. “We may not have the complete picture of that patient’s healthcare encounters,” Dr. Curtis said.
EHRs from a single health system typically capture 50% or more of patient encounters for fewer than 30% of patients, she said. This may not be an issue for patients with stable health data but exposures and outcomes that are occurring in different healthcare systems aren’t being captured and will affect study outcomes.
Additionally, such data as demographics and procedures may be standardized within EHRs, but vitals, lab results and symptoms may not be easy to come by. This can be true even if natural language processing is used to go through the data.
By comparison, claims data from a private or public insurer may have better structured, standardized data. However, reimbursement can influence coding, and clinical detail may be limited.
Claims data from private or public insurers may also be incomplete. “About 15% of individuals change health insurance in a given year, and 20% or more spend one month uninsured each year,” Dr. Curtis said. Each year, for example, about 25% of Medicaid beneficiaries change coverage or experience a gap in coverage. All of this can lead to data gaps.
Combining data from EHRs and insurance claims may seem like a reasonable way to try to address these limitations. However, with the exception of Medicare data for those 65 and older, the reality is much more complex, Dr. Curtis said.
She shared the example of a study of heart failure patients at Duke, in which researchers assumed that most patients received care at that institution. Researchers identified 20 distinct payers and noted that 80% of patients were covered by nine payers. “This gives you a sense of the linkage that may be required,” she said.
Linkage using claims data also ignores uninsured patients, who may have complex health issues.
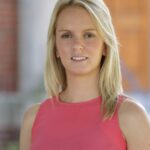
Dr. Bradley
Choosing fit-for-purpose data sources, managing treatment changes over time and considering relevant risk windows are also important considerations to optimize post-marketing safety assessments, said Marie Bradley, PhD, MPharm, MScPH, senior epidemiologist with the Division of Epidemiology, Office of Surveillance and Epidemiology, Center for Drug Evaluation and Research with the FDA.
Like Dr. Curtis, Dr. Bradley shared some of the benefits and risks associated with various data sources, such as EHRs, insurance claims and registries. For instance, registries may be rich in clinical data but often have small, highly selected patient groups and a lack of comprehensive data on drug use.
EHRs may contain more accurate information on diagnoses than other data sources and often contain data on smoking status or body mass index. Body mass index may be an important confounder in, for example, studies examining certain cancers or diabetes as outcomes.
Connecting Outcomes to Treatments
Deciding how to handle patients who switch treatments after initiation is another concern, Dr. Bradley said. This often arises for rheumatology treatments, and there are a few possible ways to manage this in a study. Using an as-treated approach, researchers can censor or suppress data from patients who switch treatments to ensure that only outcomes occurring while the patient is on the study treatment are attributed to the drug. This avoids questions about whether a new outcome is associated with the initial treatment or as a result of a newer treatment. One challenge to keep in mind is that a large degree of censoring can lead to selection bias, sample size concerns and a study population restricted to those likely to do well on the treatment (i.e., those likely to have a good response and no adverse events).
Another approach is intention to treat, which does not raise selection bias issues but could lead to increasing misclassification of exposure over time. In a prospective randomized study, this approach includes all participants in the statistical analysis; they are analyzed according to the group they were originally assigned, regardless of what treatment (if any) they received.
The timing of study measurements is also important. Study outcomes may not occur immediately after drug initiation, and some drugs used to treat rheumatic conditions may have latent effects after discontinuation. It can be useful to define a latent period after drug initiation in which outcomes are not counted, as well as an extension period after discontinuation—perhaps 6 to 12 months depending on the study and treatment—to account for latent effects, Dr. Bradley said.
Other challenges include identifying true new users of a drug, confounding by indication and choosing an active comparator for studies in the real world.
Vanessa Caceres is a medical writer in Bradenton, Fla.