‘I think imaging is the entrance door for AI in rheumatology.’ —Thomas Hügle, MD, PhD
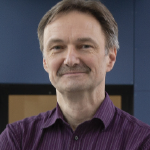
Dr. Stoel
For some situations, says Dr. Stoel, automation is necessary: for example, when the amount of imaging data is beyond what can be assessed manually or to detect subtle differences in brightness (i.e., intensity) not accessible to the human eye.
This last point—limits of the human eye to detect differences in imaging intensities—has spawned a separate field of AI research. Called comparative imaging, this area of AI is focused on providing the radiologist or rheumatologist with different images to help them find locations of—for example—progression or regression of joint disease associated with RA.
Examples of work being done in this area include software Dr. Stoel and his colleagues have developed that can show inflammatory changes in vertebral lesions in axial spondyloarthritis, as well as software developed to map local changes in lung density over time in patients with systemic sclerosis.
These are some of the basic ways in which AI can be used in rheumatology to enhance imaging interpretation. Dr. Stoel also mentioned limitations, such as the need for high-quality inputs to generate valid algorithms, the need for human expertise when developing the automated design for neural networks, the need for strict quality control of training, test and validation sets, and proper optimization of the imaging protocol.
Dr. Stoel thinks it unlikely that AI and machine learning will replace humans, but that a hybrid scenario, using both human and artificial intelligence, will be the most likely paradigm going forward.
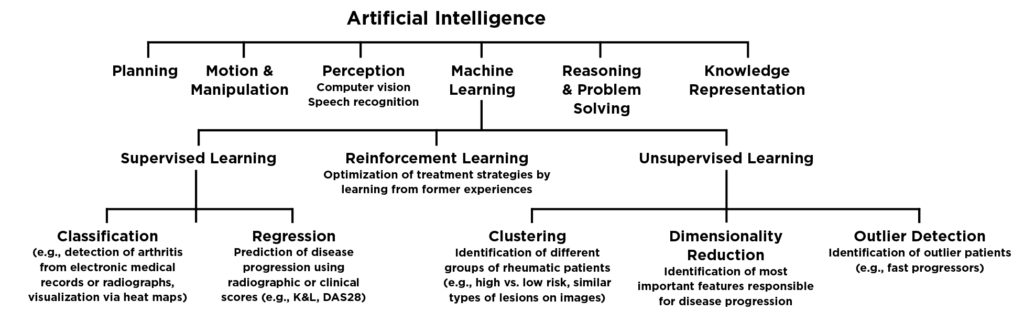
Figure 1. Created and copyright by Thomas Hügle; printed with permission
Change Is Coming
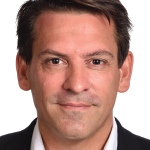
Dr. Hügle
In recognition of the importance of big data and such tools as AI and machine learning to the practice of rheumatology, the European League Against Rheumatism (EULAR) published recommendations to create a framework for how to apply big data in rheumatic and musculoskeletal diseases.5 According to EULAR, big data provides unprecedented opportunities that can deliver transformative discoveries in research and practice. The ultimate goal of using big data is to improve the health, lives and care of people, but ethical issues related to transparency, identity, confidentiality and privacy must be taken into consideration.
Prof. Laure Gossec, a rheumatologist at Sorbonne University, Paris, and the lead author of the EULAR recommendations, emphasizes that big data is coming. “It will change the way we perform studies, and also within a few years the way we diagnose and treat patients,” she says. “The methods are quite different from the usual analyses, and rheumatologists need to be aware of this big change coming up.”
As rheumatologists, like all clinicians, become more familiar with AI and machine learning and its capabilities, it is important to understand the benefits and limitations to ensure appropriate use of these sophisticated tools.
Dr. Hügle says AI will support and empower physicians. “There is much room for improvement in patient care in rheumatology,” he says. “If doctors have more time for interaction and difficult tasks and if a patient gets an automated second or third opinion, that’s a good thing.”