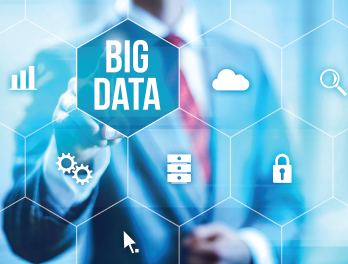
Mikko Lemola / shutterstock.com
How will big data mined from huge sample sizes in research cohorts, electronic health records, personal health data (e.g., heart rates from Fitbits) and insurance claim data sets change the way physicians interpret something as simple as complete blood count (CBC) test results for individual patients? According to the authors of a paper in the May 15 issue of the Journal of the American Medical Association, big data may change the definitions of normal or healthy patients used for comparison in research studies and clinical trials, how we interpret individual patient’s lab test results and even the concept of good health itself.1
When physicians interpret many laboratory test results (such as CBC), they often consider the patient’s age, race, ethnic ancestry or sex, the co-authors of “In the Era of Precision Medicine and Big Data, Who Is Normal?” write. In addition, physicians interpret results in reference to standard ranges determined in research studies, and these ranges are typically based on results from small sets of normal or healthy individuals who are demographically similar. However, as physicians utilize data gathered from large-scale genomic studies, they will compare patients’ test results to a normal reference population that is increasingly specific. How will this more granular approach affect medical practice and, potentially, patient outcomes?
Large Data Sets
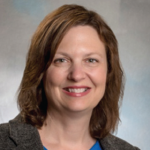
Dr. Karlson
Precision medicine and large-scale sample sizes will reshape our concepts of normality and health because “as we have more and more big data, more people are exposed to more information about themselves and their health that they try to interpret and place into context,” says co-author John P.A. Ioannidis, MD, DSc, professor of medicine and health research and policy at the Stanford Prevention Research Center at Stanford University in Stanford, Calif. They must decide if results are normal or if they should do something about it. However, as precision medicine becomes more practical and commonplace, physicians, including rheumatologists, should “be aware of our difficulty to define what is normal and what is abnormal, and don’t overreact. We should not end up treating numbers in massive scale,” says Dr. Ioannidis.
Large data sets that help drive precision medicine allow “study of stratified variation and clinical outcomes at scale,” according to the paper. Now that researchers are no longer limited to small sample sizes in studies, it is more challenging to define the normal or healthy population. As the definition of the normal patient population for any given condition becomes more granular, it is important to define “who is normal” to accurately interpret test results, the researchers say.
‘Imagine 1 million samples! The goal is to genotype & phenotype all of them.’ —Dr. Karlson
Changing Reference Ranges
Dr. Ioannidis and his co-authors used hemoglobin A1c (HbA1c) as an example of a common blood test result that can be reinterpreted through big data. HbA1c was recently found to underestimate past glycemia in African-American patients with the sickle cell trait, but researchers have yet to determine whether “more granular stratification” of populations with sickle cell matches up with clinical outcomes.2 Big data sets may make this more feasible, but it’s important to properly define normal test result ranges, they write. The Clinical and Laboratory Standards Institute’s guidelines, published in 2010, recommend that 120 reference individuals be used to establish normal intervals for many test results, but many studies use a smaller number to verify reference ranges, they add.3