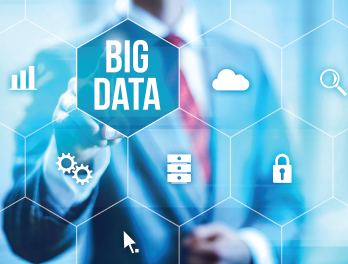
Mikko Lemola / shutterstock.com
How will big data mined from huge sample sizes in research cohorts, electronic health records, personal health data (e.g., heart rates from Fitbits) and insurance claim data sets change the way physicians interpret something as simple as complete blood count (CBC) test results for individual patients? According to the authors of a paper in the May 15 issue of the Journal of the American Medical Association, big data may change the definitions of normal or healthy patients used for comparison in research studies and clinical trials, how we interpret individual patient’s lab test results and even the concept of good health itself.1
When physicians interpret many laboratory test results (such as CBC), they often consider the patient’s age, race, ethnic ancestry or sex, the co-authors of “In the Era of Precision Medicine and Big Data, Who Is Normal?” write. In addition, physicians interpret results in reference to standard ranges determined in research studies, and these ranges are typically based on results from small sets of normal or healthy individuals who are demographically similar. However, as physicians utilize data gathered from large-scale genomic studies, they will compare patients’ test results to a normal reference population that is increasingly specific. How will this more granular approach affect medical practice and, potentially, patient outcomes?
Large Data Sets
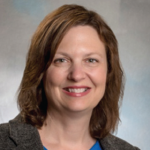
Dr. Karlson
Precision medicine and large-scale sample sizes will reshape our concepts of normality and health because “as we have more and more big data, more people are exposed to more information about themselves and their health that they try to interpret and place into context,” says co-author John P.A. Ioannidis, MD, DSc, professor of medicine and health research and policy at the Stanford Prevention Research Center at Stanford University in Stanford, Calif. They must decide if results are normal or if they should do something about it. However, as precision medicine becomes more practical and commonplace, physicians, including rheumatologists, should “be aware of our difficulty to define what is normal and what is abnormal, and don’t overreact. We should not end up treating numbers in massive scale,” says Dr. Ioannidis.
Large data sets that help drive precision medicine allow “study of stratified variation and clinical outcomes at scale,” according to the paper. Now that researchers are no longer limited to small sample sizes in studies, it is more challenging to define the normal or healthy population. As the definition of the normal patient population for any given condition becomes more granular, it is important to define “who is normal” to accurately interpret test results, the researchers say.
‘Imagine 1 million samples! The goal is to genotype & phenotype all of them.’ —Dr. Karlson
Changing Reference Ranges
Dr. Ioannidis and his co-authors used hemoglobin A1c (HbA1c) as an example of a common blood test result that can be reinterpreted through big data. HbA1c was recently found to underestimate past glycemia in African-American patients with the sickle cell trait, but researchers have yet to determine whether “more granular stratification” of populations with sickle cell matches up with clinical outcomes.2 Big data sets may make this more feasible, but it’s important to properly define normal test result ranges, they write. The Clinical and Laboratory Standards Institute’s guidelines, published in 2010, recommend that 120 reference individuals be used to establish normal intervals for many test results, but many studies use a smaller number to verify reference ranges, they add.3
Rheumatologists also consider such factors as age when interpreting some lab test results, such as erythrocyte sedimentation (sed) rate or C-reactive protein (CRP), says Elizabeth W. Karlson, MD, senior physician and leader of the Rheumatic Disease Epidemiology Group at Brigham and Women’s Hospital, Boston.
“Every clinician is taught to think about age when looking at those results. Interpreting a high sed rate is different if a patient is 80 instead of 40,” she says. Big data may not change established cutoffs for antibody tests, such as rheumatoid factor or anti-cyclic citrullinated protein antibodies (ACPA), but these ranges were based primarily on studies of mainly white patients, she says. “Past studies probably used blood banks for samples. Now, we are using large-scale biobanks, which have a more diverse representation of age, ethnicity and other factors.”
Most large-scale genome-wide association studies (GWAS) also mainly used data from white people, says Dr. Karlson, but genetic association can differ by race/ethnicity for some variants. To diversify genomic studies, she and other scientists are working on the All of Us Research Program, a new nationwide biobank research program that is part of the National Institutes of Health’s Precision Medicine Initiative. All of Us includes more than 120 recruitment sites across the country, and its goal is to recruit 1 million or more diverse participants of many ages, races, ethnicities and living environments reflecting groups who have been traditionally under-represented in biomedical research. Participants undergo physical examinations, answer health questions and contribute blood and urine samples.
Dr. Karlson directs one of the recruitment sites, All of Us New England, which has recruited more than 4,000 people in metropolitan Boston so far. Nationally, 81,000 people have signed up for the program, and 40,000 people (including 46% from racial and ethnic minorities and 76% from other under-represented groups) have completed all the steps, she says.
“Imagine 1 million samples! The goal is to genotype and phenotype all of them,” Dr. Karlson says. “The principle of this program is that all participants can see their test results and data over time, learn how to use their data and hear what research is being done. I think the transparency is contributing to incredible rates of participation in underrepresented communities.”
Imprecise Definitions
Health lacks a universally accepted definition, Dr. Ioannides and his co-authors write. In the Centers for Disease Control and Prevention’s National Health and Nutrition Examination Survey (NHANES), conducted from 2013–14, researchers analyzed survey data using three definitions of normality or health: 1) absence of common diseases such as heart disease or cancer, 2) a patient’s self-rating of health as excellent overall, and 3) the inclusion of only patients aged 18–40. Many patients who met one definition would be considered abnormal if they failed to meet one of the others, and only 5% of the NHANES survey population met all three, the paper says.
What should the ordinary clinician keep in mind about normality when reviewing a patient’s labs? “Try to understand what each claimed abnormality means, and whether it deserves any action,” says Dr. Ioannidis.
Big data culled from electronic health records (EHRs) and insurance claims may provide some solutions to these challenges, they write. If longitudinal outcomes data can be reliably linked at the individual level, researchers could test the clinical importance of differences in reference intervals. Shared databases may allow researchers to analyze across big data sets and account for the scale of multiple testing. Definitions of normal reference ranges for common test results could be customized based on the individual patient’s age, race and other attributes, and a physician could access this information through the EHR right at the point of care. Genetic ancestry data, which is becoming more easily available, could be paired with this data as well, they write.
More diverse patient representation in All of Us will generate big data that leads to more refined, precise care for patients with rheumatic diseases, says Dr. Karlson. She and the co-authors of the new paper share a vision of genomic data analyses being delivered to the treating physician at the point of care.
“As we look at markers in patients’ blood, we will have better reference ranges and know how to more accurately interpret them,” she says. “Care will be more customized. We will consider the person’s age or sex, and ask, ‘Is the ANA [anti-nuclear antibody] cutoff different for women than men, or does it vary by race and ethnicity? You may know the answer to that question in your mind, but it has not been part of the EHR report. This way, a physician doesn’t need vast knowledge of every test. This data could be customized for every patient. I don’t necessarily see that we’re ready to do that for genetic data now, but in the future, every patient could be genotyped.”
Genetic risk scores for various conditions could be used to manage care and screenings years before active disease develops, she says.
When All of Us New England was developed, “we had that exact same question of ‘What is normal?’” says Dr. Karlson. “We need to think about terms like ‘resilience’ instead, and maybe come up with a new term instead of a word like ‘normal.’ We need to learn what makes certain people resilient to developing chronic diseases in their life.”
Susan Bernstein is a freelance medical journalist based in Atlanta.
References
- Manrai AK, Patel CJ, Ioannidis JPA. In the era of precision medicine and big data, who is normal? JAMA. 2018 May 15;319(19):1981–1982.
- Lacy ME, Wellenius GA, Sumner AE. Association of sickle cell trait with hemoglobin A1c in African Americans. JAMA. 2017 Feb 7;317(5):507–515.
- Horowitz GL, Altaie S, Boyd JC, et al. EP28-A3C: Defining, Establishing, and Verifying Reference Intervals in the Clinical Laboratory, 3rd Edition. CLSI, 2010.