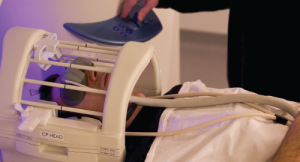
Mark Harmel / Science Source
Brain imaging can distinguish fibromyalgia patients from healthy controls with high sensitivity and specificity, according to two papers published nearly simultaneously in Pain late last summer, by groups at the Universities of Colorado and Michigan, respectively.
Somewhat surprisingly to the authors and others, in the Colorado study, which used both painful and nonpainful stimuli, the latter produced the strongest signals. If validated, the research may lead to a better understanding of fibromyalgia and improved treatments.1
“Both of these papers highlight the importance of sensory processing regions in experiencing chronic pain in fibromyalgia,” says Yvonne Lee, MD, MMSC, assistant professor at Harvard Medical School and Brigham and Women’s Hospital, who was not involved in the research. “Regulation of chronic pain may occur not only through classic pain pathways, but also via processes that affect sensitivity to a wide variety of stimuli.”
In the Colorado study, the first to be published, the investigators, including Tor Wager, PhD, and Jesus Pujol, MD, used fMRI to study the brain activity of 37 fibromyalgia patients and 35 healthy controls, as they were exposed simultaneously to various nonpainful visual, auditory and tactile clues (multisensory stimulation), and separately to painful pressure. Dr. Wager is professor of psychology and neuroscience, and director of the Cognitive and Affective Neuroscience Laboratory at his institution.
The multisensory stimulation included simultaneous exposure to flashing checker boards that alternated the positions of the white and black squares six times per second and auditory stimulation from each of 15 tones. At the same time, subjects were instructed to touch the tip of their right thumb to the fingers on that hand in rapid succession. “This approach allowed us to maximize signal power and challenge both sensory and motor systems efficiently,” the investigators write.
Nonpainful Stimuli Discriminate Better
The resulting data were analyzed using machine learning, a cutting-edge methodology that was applied to neuroimaging data to identify whether a person was a patient or a healthy control. Machine learning is a type of artificial intelligence that provides computers with the ability to learn without being explicitly programmed to do so. In this particular case, the investigators used a type of machine learning that Dr. Wager described as “a family of algorithms for finding patterns in complex data and using them to make accurate predictions.” It identified the patterns of brain activity with the biggest differences between patients and controls, distinguishing them with a 93% success rate.