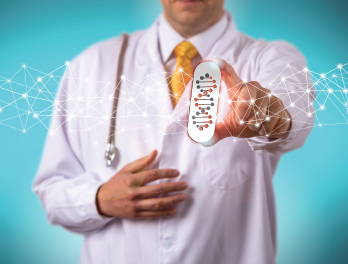
LeoWolfert / shutterstock.com
SNOWMASS VILLAGE, COLO.—Choosing the right treatment at the right time is the brass ring all rheumatologists hope for. Precision medicine provides the ability to leverage clinical, biomarker and omics data to predict and personalize future treatment for rheumatoid arthritis (RA).
“New data and new methods to analyze the data are helping us better predict patterns of disease and treatments that work well for subgroups of patients,” noted Jeffrey Curtis, MD, MS, MPH, during his presentation on precision medicine in rheumatology at the 2020 ACR Winter Symposium in January. Dr. Curtis is the Harbert Ball Professor of Medicine in the Division of Clinical Immunology & Rheumatology, University of Alabama at Birmingham (UAB). He is the co-director of the UAB Pharmacoepidemiology Unit and has led the clinical trials unit at UAB for more than a decade, with a focus on RA and psoriatic arthritis and spondyloarthritis.
Predicting RA Outcomes
Early indications are that some prediction models may be accurate enough for clinical care and management. But Dr. Curtis asked, “How good a prediction is good enough for clinicians?”
Dr. Curtis previously conducted research to predict low disease activity and remission using early treatment response to anti-tumor necrosis factor therapy in patients with RA using data from an etanercept trial (TEMPO). He said the results of that trial indicated an accurate prediction could be made for 80–90% of patients within 12 weeks of starting therapy about whether they were likely to have low disease activity at week 52. However, researchers needed to see the remaining 10–20% of patients following additional time on therapy to determine whether treatment should continue.1
Subsequent investigations have looked at predicting treatment response earlier (e.g., as early as four weeks after starting therapy). The ability to predict treatment response before a patient starts therapy based on blood or genetic-derived factors remains the holy grail for researchers.
He discussed balancing rigor (such as focusing on low disease activity, if not remission as the optimal target) and practicality (e.g., settling for significant improvement), and maximizing predicted response vs. non-response in assessing treatment efficacy.
In research to predict response to biologic drugs, one growing area of study is the identification of immunological biomarkers of treatment response through such methods as evaluating synovial tissue and fluid. Various biomarkers produced by a range of cell types have been suggested to be important. For example, macrophages in the synovial lining of the joint are important elements of RA pathogenesis, and macrophage-derived markers have been correlated with future treatment response.
One study used single-cell RNA-sequencing to uncover four distinct subsets of mouse synovial macrophages and identified a dynamic role for synovial macrophages in inflammation.2
Dr. Curtis stressed the accuracy of prediction models should be judged by clinicians to consider maximum benefit in real-world application.
Sharing Data
An important goal with precision medicine is to collect actionable, relevant data and share it in a fashion that improves the clinical and research community. Dr. Curtis suggested clinical data registries, such as the ACR’s Rheumatology Informatics System for Effectiveness (RISE) registry, can be valuable by providing rheumatologists with the ability to compare specific aspects of a patient’s disease and response to treatment with similar patients treated differently who may live across the county. Understanding what factors relate to not changing therapy is likewise important.
For example, an analysis including treatment escalation data from the ACR RISE registry by Dr. Curtis and colleagues showed one‐third to two‐thirds of RA patients failed to change their treatment, even when the patients remained in moderate or high disease activity.3 Dr. Curtis and his co-authors concluded that multi-modal interventions developed and tested among RA patients and their providers could be beneficial in supporting shared decision making and goal‐directed care to remove barriers to escalating treatment. Reducing inappropriate practice-level variability and better engaging patients in driving their own healthcare are valuable roles for this use of big data.
Consider the Patient Perspective
In work to personalize treatment, Dr. Curtis stressed the importance of acknowledging that patients can have very different goals for their care. “A patient’s treatment goals and enthusiasm to improve their health condition may depend on their appetite to switch therapies. Sometimes, this will entail undertaking some risks associated with treatment change, and not all patients will agree to that.”
To understand the patient’s perspective in their care trajectory, rheumatologists need to facilitate shared decision making to understand the patient’s goals, and “this takes time and a dialogue,” he cautioned.
Dr. Curtis noted that whether you are striving to understand a patient-reported outcome or working with patients to set goals, the point is to make sure your patients understand that you care about them as a whole patient, “not just inflamed joints and skin.”
He went on to share developments in efforts to redesign patient-reported outcome-data capture using such tools as the National Institutes of Health (NIH) Patient-Reported Outcomes Measurement Information System (PROMIS) to evaluate and monitor physical, mental and social health in adults and children. The NIH PROMIS system is a set of efficient patient-reported outcome data collection tools to capture what matters most to patients. These data can be used to assess a patient’s health status, physical function, mental health and ability to engage in such activities as spending time with friends or engaging in hobbies at home. They also measure a patient’s perception of pain, anxiety and fatigue.
Dr. Curtis and colleagues applied PROMIS instruments (questionnaires) to assess treatment response in the AWARE trial. He and his co-authors found high correlation between PROMIS instruments and the traditional patient and physician assessments among more than 1,000 RA patients starting a new infusion biologic.4
These results support the validity of using the NIH PROMIS system to assess outcomes that matter to patients. Moreover, this can be done at home and captured electronically (e.g., on a smartphone), and is highly efficient, taking less than one minute per domain. Capturing patient-reported outcomes in this fashion is a tremendous improvement over the traditional methods that patients don’t care much about (e.g., counting the number of swollen and tender joints).
Carina Stanton is a freelance science journalist based in Denver.
References
- Curtis JR, Yang S, Chen L, et al. Predicting low disease activity and remission using early treatment response to antitumour necrosis factor therapy in patients with rheumatoid arthritis: exploratory analyses from the TEMPO trial. Ann Rheum Dis. 2012 Feb;71(2):206–212.
- Montgomery A, Chen S, Homan P, et al. Single cell RNA-sequencing reveals distinct macrophage subsets in the joint with differing ontogenies during steady-state and arthritis [abstract]. Arthritis Rheumatol. 2019;71(suppl 10).
- Yun H, Chen L, Xie F, et al. Do patients with moderate or high disease activity escalate rheumatoid arthritis therapy according to treat‐to‐target principles? Results from the Rheumatology Informatics System for Effectiveness registry of the American College of Rheumatology. Arthritis Care Res (Hoboken). 2020 Feb;72(2):166–175.
- Bingham C, Kafka S, Black S, et al. Construct validation of PROMIS short form and profile-29 T-Scores with SF-36 in rheumatoid arthritis patients treated for 1 year: Results from a real world evidence-based study in the United States [abstract]. Arthritis Rheumatol. 2019;71(suppl 10).