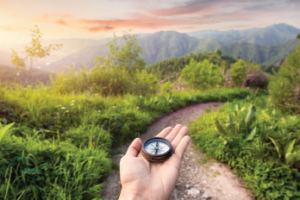
Pikoso.kz / shutterstock.com
I nodded, gravely.
I was recently privileged to attend a symposium on eosinophils. I realize that this sounds odd. And it was odd, mainly because I’m not an eosinophil expert. Still, they were in need of someone who knew something about eosinophilic granulomatosis with polyangiitis, so I managed to sneak in through the back door.
As an aside, I think we are on the cusp of a revolution in how we think about diseases mediated by eosinophils. Although we treat them as distinct diagnoses, a tremendous amount of clinical overlap exists between eosinophilic granulomatosis with polyangiitis and other diseases mediated by eosinophils (e.g., chronic eosinophilic pneumonia, eosinophilic esophagitis and other idiopathic hypereosinophilic syndromes). Interestingly, all of these syndromes seem to respond to therapy directed against interleukin 5, regardless of what you call them. Eventually, mepolizumab, reslizumab, benralizumab and other such drugs may unify these diagnoses, in the same way that rituximab has brought together idiopathic orbital pseudotumor, Mikulicz syndrome and idiopathic pancreatitis under the rubric of the IgG4-related diseases.
We are still at just the early stages, however, of understanding diseases mediated by hypereosinophilia. Because of this, at this symposium, we were given a task: propose what we would need to make a breakthrough. Unlike in most grant brainstorming sessions, we were specifically instructed not to consider the cost.
As you could imagine, our proposal quickly spiraled from unlikely to ludicrous. First, we needed substrate: knowing the environment can stimulate eosinophils, we proposed collecting samples from all continents, from patients at all decades of life. We then had to figure out how to characterize these samples. After some back and forth, one of the PhDs in the group exclaimed, “Drop-seq!”
I nodded, gravely.
I was nodding to disguise the fact that I had no idea what he was talking about.
Recently, I’ve been doing a lot of that sort of grave nodding. Seq in these cases stands for sequencing, and the suffix seems to be making its way into a lot of conversations. I know that techniques like PhIP-seq and Drop-seq have dramatically sped the process of discovery. But what do they mean?
Autoantibody Discovery
Let me know when the antibodies come back. As a consultant, I have spoken these words hundreds of times. Autoantibodies are so central to how we think about rheumatic disease, both in terms of classification and pathogenesis, that I am generally loathe to make a final diagnosis if that information is not yet firmly in hand. But how did we come to identify these autoantibodies in the first place?
Consider, for example, mixed connective tissue disease. Undifferentiated connective tissue disease is the catchall label we give patients we suspect have some autoimmune phenomenon that has not yet been given a proper name. Mixed connective tissue disease was first described by Sharp et al. in 1972, when he identified 25 patients who had features of systemic lupus erythematosus, scleroderma and polymyositis.1 Sharp noted these patients were unified by the presence of antibodies against ribonucleic protein (RNP). He described the process of identifying the presence of these anti-RNP antibodies thus:
To study the specificity of antibodies … enzymatic digestion studies were performed. Treatment of the ENA antigen on the red cells with RNase completely eliminated or markedly reduced the agglutination reaction with the patient’s serum in every case of mixed connective tissue disease whereas treatment with trypsin reduced the titer to some extent. Treatment with DNase had no effect. … [T]he speckled pattern of the antinuclear fluorescence was eliminated by treatment of tissue sections with RNase but not DNase prior to incubating them with serum from patients with mixed connective tissue disease.
In other words, they conducted a series of experiments to narrow the biochemical properties of the autoantigen. But they also got lucky: The autoantigen just happened to be something that could be affected meaningfully by the reagents they had available.
This observation is not meant, by any means, to denigrate the value of their work. Happenstance is often a valuable partner in discovery. There is no particular reason, for example, that antibodies that recognize proteins in the cytoplasm of neutrophils should be associated with glomerulonephritis, but the discovery of anti-neutrophil cytoplasmic antibodies (ANCA) must be one of the most important advances made in our understanding of the systemic vasculitides.2 Similarly, a breakthrough in our understanding of immune-mediated necrotizing myopathies came from the recognition that autoantibodies from some of these patients bound to a protein that was the same molecular weight as 3-hydroxy-3-methylglutaryl coenzyme A reductase (HMGCR), an enzyme better known to you as the pharmacologic target of statins.3
The right insight, with the right reagent, at the right time. These are the classic ingredients for autoantibody discovery. And with these ingredients alone we would undoubtedly continue to lurch toward discovery, occasionally achieving new insights, interspersed with long periods of frustrating silence.
Identifying the presence of a specific autoantibody when you have a specific target in mind is not difficult: an autoantibody target (generally, a protein) is bound to the bottom of a plate. Serum from the patient is introduced, and you see if the patient has an antibody that binds to the target. This, in a nutshell, is how the enzyme-linked immunosorbent assay (ELISA) works and is the foundation of the diagnostic antibody testing that keeps Quest Diagnostics and LabCorp in business.
Identifying a novel autoantibody when you don’t know what the target might be is more difficult. If you were using ELISA, you could methodically work your way through every target, one by one. Of course, empires would rise and fall in the time it would take to search through the more than 20,000 proteins manufactured by the human genome.4
Enter PhIP-seq.
One advance in autoantibody discovery came through the use of viruses known as bacteriophages. A phage-display library incorporates human DNA into the viral genome, allowing various human peptides to become part of the viral coat.5 Picture a football stadium in which all the fans were asked to hold banners high over their heads so the cameras could get a good look at each sign. Now, instead of banners, picture every fan holding a small protein fragment; instead of a camera, picture an antibody searching for its target. This is basically how a phage display library works.
The problem with this technique is that not every bit of DNA will be correctly incorporated by the viral genome. Therefore, the proteins expressed by the viruses often represent an incomplete version of reality. Even under the best of circumstances viruses can display only fragments of proteins, not complete proteins with their tertiary structures intact. It’s like reading a sonnet when not every word is successfully printed on the page—you may still see enough to get the gist, or you may just completely miss the point.
Phage immunoprecipitation sequencing (PhIP-seq) uses viruses to display the peptidome: a close-to-complete set of protein fragments encoded by the human genome.6 Because the peptidome uses protein fragments and not complete proteins, not every human autoantibody will be able to find an appropriate target, even with this system. But it allows us to get much closer to this goal than was previously possible.
Of course, this one sentence belies the tremendous complexity of the work that follows: Once an antibody binds a protein, the antecedent DNA must be amplified, then sequenced, to identify the target. But it is not hard to see how this technique could open doors previously slammed shut.
Drop-Seq & the Problem of Specificity
Everything we have discussed up to this point has to do with autoantibody discovery. But if we want to learn more about the cause of disease, we must look at the transcriptome.
If DNA can be thought of as the Book of Life, then RNA represents the words read off the page. As anyone who studied biology in college can tell you, DNA produces messenger RNA, which serves as the template for the production of proteins. The transcriptome is the sum total of all messenger RNAs expressed by an organism. Characterizing the transcriptome—and how the transcriptome changes in the journey from health to illness—may be key to understanding human disease.7
Easier said than done. Consider the kidney, for example. Let’s say that you are enrolled in a biology seminar, and for your final exam, I ask you to propose an experiment to learn more about glomerulonephritis. You may propose to take a kidney biopsy and look at the transcriptome to determine how inflammation changes the proteins expressed by the kidney.
Unfortunately, that may not be good enough. Every kidney biopsy actually consists of multiple cells—endothelium, epithelium, leukocytes, stromal cells—each of which expresses the proteins that serve its needs. What you really would want is to be able to analyze the transcriptome of every cell, individually.
Enter Drop-seq.
First, I should admit that Drop-seq has nothing to do with PhIP-seq, other than being shorthand for the new generation of multiplexed technologies, which perform hundreds of tests in parallel. These high-throughput screening technologies accelerate discovery by performing multiple assays simultaneously.8 It’s almost like having a hundred grad students in your lab, each of whom is performing a slightly different version of the same experiment all at the same time.
With Drop-seq, every cell from a tissue sample is placed in a bubble of fluid, which serves as a miniature lab for that single cell. Using this technique, Steven A. McCarroll’s lab was able to analyze the transcriptome of over 44,000 mouse retinal cells, and identified 39 distinct populations.9 Of these populations, you could imagine that some are vital to our understanding of disease states, and some of them are completely irrelevant. But at least now we have a fighting chance of telling them apart.
Just like PhIP-seq opens new doors for autoantibody discovery, single-cell genomics, transcriptomics (like Drop-seq) and proteomics may open new doors for drug discovery. The Accelerating Medicines Partnership (AMP) is betting big on this. Created in 2014, it is a consortium that includes the NIH, the FDA and multiple pharmaceutical and nonprofit organizations. The consortium recognizes the future of drug discovery lies in developing more efficient ways of determining how novel compounds affect the cells they target. They note:
The entire biomedical research community and the public have a shared interest in compressing the timelines, reducing the costs, and increasing the success rates of new targeted therapies. Given the amount and complexity of the data, this goal will require a systematic approach in which government, academia, industry, and patient groups work collaboratively to sift through the flood of disease targets and find the ones most likely to prove responsive to treatments.10
I think I’m starting to understand why these technologies have so many people so excited. Thinking back to the symposium I attended, it is easy to see how Drop-seq, for example, would be invaluable to expanding our understanding of hypereosinophilic disorders. It would allow us to see the difference in the transcriptome of eosinophils from a patient with eosinophilic granulomatosis with polyangiitis, and compare it to the transcriptome of a patient with allergic sinusitis or eosinophilic colitis. We would finally have a way of understanding these diseases that is more sophisticated than counting the number of eosinophils that appear on a microscope slide. Similarly, PhIP-seq could be used to identify novel autoantibodies that drive all of these diseases.
These are not the only seq technologies that are changing the landscape of discovery. There are also Epi-seq, CEL-seq2, MAUI-seq, ChIP-seq, Perturb-seq and others, each of which takes an older technology and makes it work faster. All of these technologies will likely change how we think about many of our diseases, making connections we don’t even know we should be looking for. Of course, these technologies come with barriers of their own: The cost of these technologies is enormous, and analyzing their output is beyond complicated. That said, up until now, research has depended on our asking questions that were answerable. Increasingly, we are now able to ask the questions we really want answered and follow the data wherever they lead.
Philip Seo, MD, MHS, is an associate professor of medicine at the Johns Hopkins University School of Medicine, Baltimore. He directs the Johns Hopkins Vasculitis Center and the Johns Hopkins Rheumatology Fellowship Program.
References
- Sharp GC, Irvin WS, Tan EM, et al. Mixed connective tissue disease—an apparently distinct rheumatic disease syndrome associated with a specific antibody to an extractable nuclear antigen (ENA). Am J Med. 1972 Feb;52(2):148–159.
- Falk RJ, Jennette JC. Anti-neutrophil cytoplasmic autoantibodies with specificity for myeloperoxidase in patients with systemic vasculitis and idiopathic necrotizing and crescentic glomerulonephritis. N Engl J Med. 1988 Jun 23;318(25):1651–1657.
- Mammen AL, Chung T, Christopher-Stine L, et al. Autoantibodies against 3-hydroxy-3-methylglutaryl-coenzyme A reductase in patients with statin-associated autoimmune myopathy. Arthritis Rheum. 2011 Mar;63(3):713–721.
- Ponomarenko EA, Poverennaya EV, Ilgisonis EV, et al. The size of the human proteome: The width and depth. Int J Anal Chem. 2016;2016:7436849.
- Bazan J, Calkosinski I, Gamian A. Phage display—a powerful technique for immunotherapy: 1. Introduction and potential of therapeutic applications. Hum Vaccin Immunother. 2012 Dec 1;8:1817–1828.
- Larman HB, Zhao Z, Laserson U, et al. Autoantigen discovery with a synthetic human peptidome. Nat Biotechnol. 2011 May 22;29(6):535–541.
- Transcriptome fact sheet. National Human Genome Research Institute.
- Inglese J, Johnson RL, Simeonov A, et al. High-throughput screening assays for the identification of chemical probes. Nature Chem Biol. 2007 Aug;3(8):466–479.
- Macosko EZ, Basu A, Satija R, et al. Highly parallel genome-wide expression profiling of individual cells using nanoliter droplets. Cell. 2015 May 21;161(5):1202–1214.
- Accelerating Medicines Partnership (AMP). National Institutes of Health.