Rheumatoid arthritis (RA) can be typed, grouped and categorized in different ways, and subgroup identification could help guide future research and treatment strategies based on which subtypes respond to which treatment. A new study explored an approach associating gene expression profiling with histologic analysis of synovium samples to define RA subtypes and then examined how these findings may integrate with each other.1
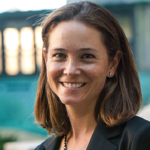
Dr. Orange
Currently, no reliable biomarkers exist to predict therapeutic response in RA patients, says physician-scientist Dana E. Orange, MD, MSc, of the Hospital for Special Surgery, New York City. Classifications derived from synovium are not yet factored into RA diagnostic criteria or treatment guidelines. Her team identified three distinct RA synovial subtypes using RNA sequencing data and applied machine learning technology to develop a method for integrating and weighing scores of histology features in a manner that predicts gene expression subtype.
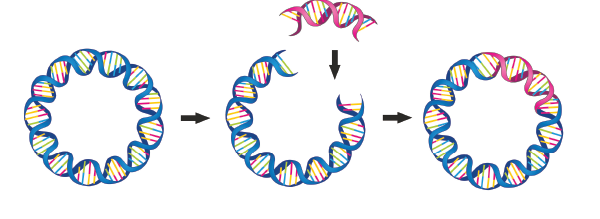
Soleil Nordicleil Nordic / shutterstock.com
The researchers examined 20 histologic features on synovial tissue samples from 123 consecutive RA patients undergoing arthroplasty at the Hospital for Special Surgery, along with six osteoarthritis patients. The study used standard hematoxylin and eosin (H&E) stain-based assay slides, a technique commonly used in medical labs, to assess histologic features.
On a subset of 45 tissue samples, they also performed gene expression profiling and identified three distinct molecular subtypes of RA: high, low and mixed inflammation subtypes. “We identified three clusters of gene expression in RA. How could we put them together when it wasn’t obvious how to associate gene expression data with histological findings?” Dr. Orange says. “They are very different types of data. That’s where we applied machine learning.”
Machine learning is a branch of computer science that uses statistical technology to “train” computers to progressively improve their performance on a specific task by breaking data down into predictive units as the analysis moves forward. Support vector machine learning uses vectors to break down the data.
In this case, the three gene expression subtypes served as labels. Support vector machine learning was used to identify the optimal weights for the histologic features allowing for prediction of the gene expression subtypes using histology data only. By using the robust RNA expression data to train the histology scoring system, the researchers could then apply their histology scoring algorithm to better interpret samples that only had histologic assessments, Dr. Orange explains. The samples with matched RNA expression and histology data were like a Rosetta Stone that enabled interpretation of the histology-only samples.