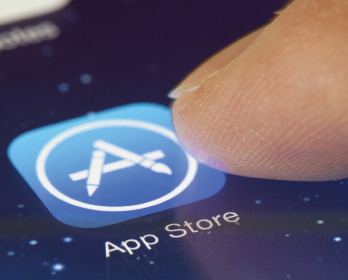
ymgerman / shutterstock.com
CHICAGO—Every minute, it seems, a new digital tool is introduced in medicine. Whether it’s a new digital measuring stick, a new data-crunching system or a new app, the tech tools form an endless convoy of options. But are they worth it? Will they really help you do your job better? Will they help patients feel better? Will they make things faster and easier? Does research back them up? A panel of experts gathered at the 2018 ACR/ARHP Annual Meeting to talk about just that.
The experts noted encouraging digital advances that appear poised to help in the realm of patient-reported outcomes, as well as to address challenges in collecting data, tracking flares, assessing medication adherence and patient education.
Patient-Reported Outcome Info
Clifton “Bing” Bingham, MD, director of the Johns Hopkins Arthritis Center, Baltimore, said PROMIS, or the Patient-Reported Outcome Measurement Information System, is showing benefits in rheumatology. This 10-year project by the National Institutes of Health, now seeing more use in clinical practice, blends advanced information technology with psychometrics and qualitative, cognitive and health survey research to create a nimbler and more usable source of patient information. It provides a more accurate picture of how patients experience their disease than such commonly used tools as the Simple Disease Activity Index, Clinical Disease Activity Index and Disease Activity Score, which tend to miss “things that patients are telling us are important,” Dr. Bingham said.
Many patient-reported outcome tools developed and used primarily in clinical trials may have limited relevance to patients seen in clinical practice and not be actionable by the provider, he said. In contrast, PROMIS measures such areas as pain, fatigue, physical functioning and emotional distress, making it relevant to many chronic and rheumatic diseases. It produces scores calibrated against the U.S. population using T-scores; the mean population score is 50 with a standard deviation of 10, so anything outside the 45–55 range is considered an outlier.
Measures may be administered with computer adaptive testing, starting with questions in the middle of the range and moving to questions positioned halfway between the latest response and the extreme on that side. Questions assessing physical function could include, “Are you able to get in and out of bed?” or “Are you able to stand without losing your balance for one minute?” This method of computer-assisted questioning allows you to obtain a precise score quickly, Dr. Bingham said. Fixed-length short forms are also available.
The scoring system places patients along a wide spectrum that doesn’t have the floor or ceiling effects that can occur with other measures, he said. For example, a paper assessing PROMIS scores found rheumatoid arthritis (RA) patients had scores all the way from 2 standard deviations worse than the norm up to 2 standard deviations better than the norm across many domains. “For each patient, the PROMIS instrument was able to identify those patients and put them at a point along the continuum,” he said.
Over time, you can look at what is normal for a given patient and then assess the change, Dr. Bingham said. “It means that [for] someone who’s a marathon runner, you can actually evaluate when they can run only five miles or when they can only walk two miles.”
A comparison of patient scores on the Modified Health Assessment Questionnaire (mHAQ) and PROMIS found that although 40% of patients scored zero on the mHAQ, indicating their health was fine, physical function scores on PROMIS tilted toward worse function, providing a truer picture of what was going on.1 PROMIS scores also have been shown to approach or even exceed normative values when patients are in remission, showing the potential rewards when a remission goal is reached.
Dr. Bingham said the group has been using PROMIS measures in clinical practice and studying the impact on patient-physician communication and decision making. “Patient-reported outcome information,” he said, “gave new information that clinicians, at the time of the visit, thought was actionable.”
Patient Data Collection Tips
Before a practice sets out to collect patient information, it must take a host of factors into account, said Eric Ruderman, MD, associate chief for clinical affairs in rheumatology at Northwestern University in Chicago. If you’re trying to collect information for use in clinical care, physician engagement is one of the most important considerations, he said. It must be a feasible endeavor, and even if it’s only a brief time commitment, its value must be clear to the clinician.
“Though four minutes isn’t a very long period of time, when you have a 20-minute office visit, that’s a big chunk of that office visit,” Dr. Ruderman said. “And if you’re going to disrupt the clinic flow in any way, you’ve got to persuade your providers … the data you’re collecting is meaningful.”
He also emphasized these factors:
- Limit data-collection effort—“You need to reduce the burden on patient and on staff” as much as possible.
- Determine the extent of the data you want to collect—Limit the complexity of the instruments to what is really necessary, because patients must be willing to participate. Will every patient have data collected every visit? Less often? Only RA patients? Only lupus patients? “We have addressed that in our practice by limiting the number of instruments we use and collecting it on every patient, every visit,” Dr. Ruderman said. “And then if the physician doesn’t find it helpful, it’s extra information. If they do, it’s useful.”
- Make it understandable—He noted the Routine Assessment of Patient Index Data 3 (RAPID3) disease activity index is used widely because it is simple and easy to understand. But however the data is presented, it must be concise and easy to use in a busy environment.
- Consider the clinic flow—When should the data be collected? If a patient shows up just a minute or two before their appointment time, there probably won’t be enough time to collect their data without delaying the physician. If it’s collected too far ahead of time, it may not be considered truly real-time data useful at a visit. The same is true if it’s collected afterward. There is no clear answer, Dr. Ruderman said.
- Monitor the process—Someone in the office must make sure the data collection is happening. This will involve reminding patients to fill out the questionnaire, collecting the information and then bringing it to the physician.
“At the end of the day, integrating all of this is the key piece,” Dr. Ruderman said, “to try to make sure it all goes in one place, so that … in practice, you can use it.”
When patients flared, researchers found, they did indeed walk less.
Predicting Flares
In another talk, Laure Gossec, MD, PhD, professor of rheumatology at Sorbonne University, Paris, discussed the way clinicians and researchers have turned to activity trackers to try to get a better handle on RA patient flares.
“They’re important for patients, but also they should be important for physicians,” Dr. Gossec said. “But we haven’t seen yet how we can collect them and make sure we’re not overdefining flares, or missing something. Questionnaires and changes in disease activity status are the usual ways to detect flares, but they don’t always agree.”
Patients’ disease activity can, of course, fluctuate wildly between visits, which provide only a snapshot in time. In a study called ActConnect, researchers turned to activity trackers on the theory that when patients are suffering a flare, they’ll walk less, because patients say activity interruption is an important aspect of their flares. In the study, 170 RA and axial spondyloarthritis patients were asked questions each week to assess flare, and their physical activity was monitored with trackers. Dr. Gossec said patients (who received the devices for free) were compliant in wearing them and in uploading their data regularly with a Bluetooth connection.
When patients flared, researchers found, they did indeed walk less. There was a significant link between physical activity and flares (P=0.03). Patients in flare tended to walk roughly 1,000 steps less per day—or about 10–15% below the normal amount of daily activity of those not in flare.
Researchers turned to a commercial source—a phone company interested in venturing into healthcare with a machine-learning angle—to analyze the data with machine learning to try to use the steps walked to predict flare. They came away with a positive predictive value of 91% and a negative predictive value of 99%.2
Dr. Gossec acknowledged the machine-learning process is a bit of a “black box.” It stands to reason, though, that the process likely involves not just the total number of steps, but the pattern of those steps—when they’re taken, skipped exercise days and pace, she said.
She said the results show it is possible to use big data analysis on rheumatology datasets. But it is not a simple proposition.
“If we want to use big data machinery to analyze things like steps per minute, we need expertise,” Dr. Gossec said. “It’s a very specific expertise. It also takes time and money.”
The ultimate use of the data is also a consideration. “Will that information go to the insurance company before it goes to the doctor?” she asked. “These are all issues we need to think about.”
Medication Adherence
Researchers are also turning to technology to monitor patients’ medication adherence, a huge but often overlooked problem, said Bernard Vrijens, PhD, chief executive officer at Advanced Analytical Research on Drug Exposure.
“When we have suboptimal adherence in practice, typically it leads to treatment failure,” Dr. Vrijens said. “When the treatment fails, the disease progresses. Sometimes we have acute events. And when the disease progresses, what we typically do is give more complex treatments. The more complex the treatment, the worse is the adherence. This is an extremely costly loop.”
Many apps push patients to take their medications, Dr. Vrijens noted. But they simply give alerts and aren’t integrated into a patient’s overall health picture. “Outcome is key. It’s not [about] taking the medication, it’s really [about] optimizing outcome, minimizing adverse effects.”
In research he has led, investigators use electronic monitoring to assess patients’ adherence—the act of a patient removing a pill from its container is recorded. The results show how vastly different patients’ adherence can be even if, overall, they have the same adherence rate.
He showed data for six patients, all with an adherence rate of 81%. But one patient tended to be diligent in the morning while faltering at night. Another started out well and then began to deviate from the schedule. Another had such wild adherence that there was no clear pattern. These details—when patients didn’t take a pill or when they took double to make up for it—are important in making treatment decisions, Dr. Vrijens said. Research also suggests showing patients their own mistakes is the best way to improve their behavior.3
Adherence has become more important lately because new therapies tend to feature a narrow therapeutic window—too little delivers little effectiveness, and too much results in toxicity, he said.
“We need to bring knowledge to the point of care” to better individualize treatment, Dr. Vrijens said. “Medication adherence is really a vital sign to measure and manage in this setting of individualization of treatment.”
Thomas R. Collins is a freelance writer living in South Florida.
References
- Bartlett SJ, Orbai AM, Duncan T. Reliability and validity of selected PROMIS measures in people with rheumatoid arthritis. PLoS One. 2015 Sep 17;10(9):e0138543.
- Gossec L, Guyard F, Leroy D, et al. Detection of flares by decrease in physical activity, collected using wearable activity trackers, in rheumatoid arthritis or axial spondyloarthritis: An application of machine-learning analyses in rheumatology. Arthritis Care Res (Hoboken). 2018 Sep 22.
- Demonceau J, Ruppar T, Kristanto P, et al. Identification and assessment of adherence-enhancing interventions in studies assessing medication adherence through electronically compiled drug dosing histories: A systematic literature review and meta-analysis. Drugs. 2013 May;73(6):545–562.