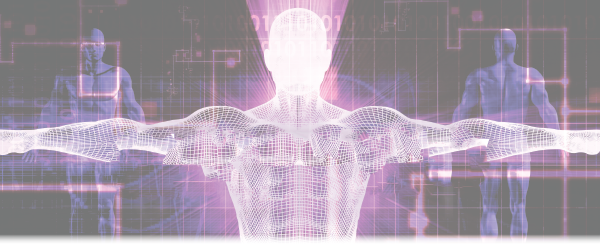
kentoh / shutterstock.com
Dr. Kim also emphasized the importance of clearly defining treatment exposures, so the two comparator groups can be presumed to be identical with respect to their risk of outcomes. This can be trickier than it seems at first. For example, it’s important to consider the concept of positivity, which states that the possibility of receiving a treatment must be greater than zero (this would not be true, for example, in case of a drug contraindication). The interventions themselves must also be well defined in areas of timeline, inclusion and exclusion criteria, and other factors.
For people intimidated by some of the details of advanced technical methods in causal inference, Dr. Kim pointed out that not all questions require such methods be employed. “Try to make the study question very simple first,” she advised. “Once you have a very straightforward causal question, start with a simpler method, and you will build up your confidence in applying advanced methods later,” she said.
For those looking to learn more about causal inference, Dr. Kim strongly recommends a free textbook by Miguel A. Hernán, MD, MPH, ScM, DrPH, and James M. Robins, MD, Causal Inference: What If, as a good entry point into the topic.1 These researchers were the first to explicitly propose a “target trial” approach to organizing an observational study, an approach also recommended by Dr. Kim.2
Target Trials
In the second part of the March 4 session, Daniel H. Solomon, MD, MPH, chief of the Section of Clinical Sciences at Brigham and Women’s Hospital and professor of medicine at Harvard Medical School, expanded on Dr. Kim’s recommendation to follow a target trial approach when designing rigorous observational studies. Dr. Solomon also serves as principal investigator on the VERITY grant and as editor in chief of Arthritis & Rheumatology.
Dr. Solomon noted that accepted research methodologies can evolve over time. For example, the Bradford Hill causal criteria have fallen out of favor somewhat as the research community has realized their limitations. In contrast, the target trial approach has been gaining momentum in recent years.
“For each observational analysis for causal inference, we can imagine a hypothetical randomized trial that we would likely prefer to conduct. That is the target trial,” says Dr. Solomon.
He noted that clinicians want their decision making to be informed by causal knowledge about comparative effectiveness and comparative safety. Deferring decisions is not really an option because it just maintains the status quo.
“A relevant randomized trial would, in principle, answer each causal question about comparative effectiveness and safety,” he said. “But we often can’t have randomized trials because they are expensive. They are sometimes unethical, depending on the study. They can be impractical, and they often can’t deliver timely answers.”
Although observational analyses cannot provide the highest level of evidence, the target trial approach provides a way to carefully and thoughtfully design observational studies, so they can provide more accurate information to inform clinical practice.
The idea is to explicitly emulate the envisioned randomized trial with observational data. This requires rigorous analysis and thorough description of various categories as they would occur in a randomized trial: eligibility criteria, treatment strategies, assignment criteria, assignment procedures, follow-up period, outcome, causal contrast of interest and analysis plan. Problems in any of these areas can lead to results that are difficult to interpret and that cannot help guide wise clinical decisions.
For example, Dr. Solomon described a systematic review he helped perform that analyzed the comparative effectiveness of different rheumatoid arthritis strategies through the lens of a target trial emulation framework.3 Such work might be very insightful, as randomized, controlled trials have been slow to fill this hole in the literature. But 29 out of the 31 trials included in their analysis had one or more design flaws, as determined by a target trial emulation approach, limiting their applicability.
Dr. Solomon sees target trial emulation as a way to strengthen causal inference and thus the strength of our confidence in found associations. “Unfortunately, with observational data, people are often a bit sloppy. That results in a lot of vagaries in the literature. We think that target trial emulation is a way of creating some rigor around how one does these comparative effectiveness studies to bring us closer to causation.”
The March 11 sessions further explored mediation analysis, a highly pertinent concept for interpreting and designing both observational and randomized trials.